티스토리 뷰
이것이 데이터 분석이다 with 파이썬 ch5-2(구매 데이터를 분석하여 상품 추천하기)
jaeyoonlee 2021. 4. 24. 20:58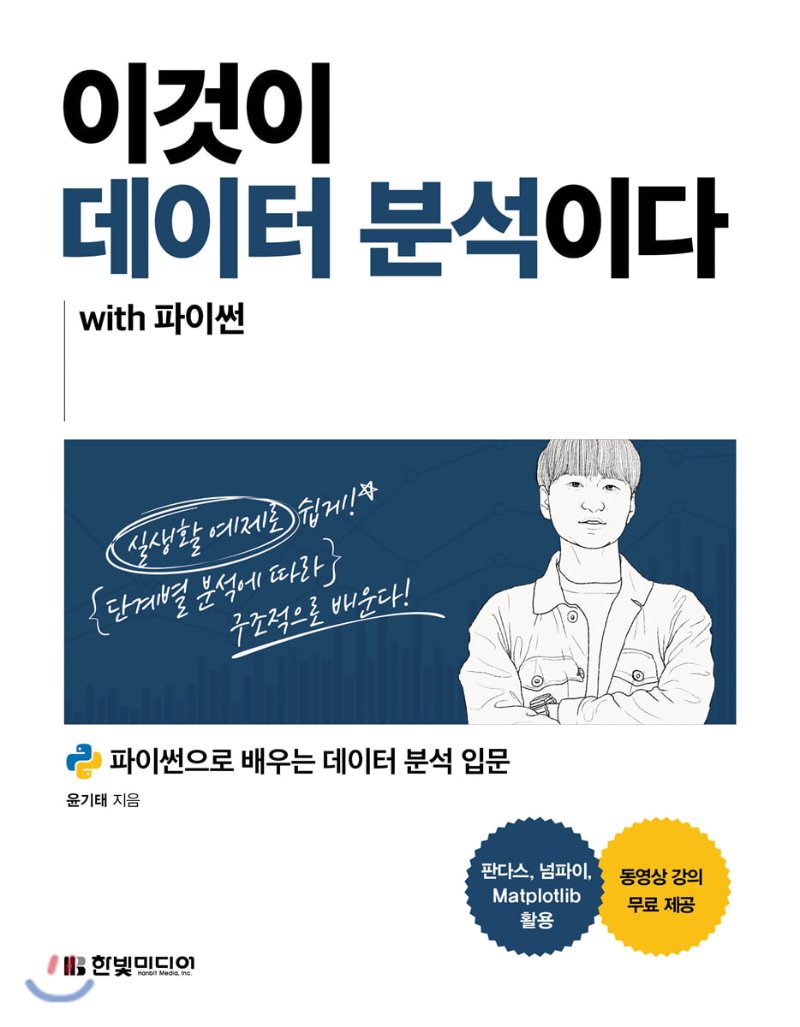
5.2 구매 데이터를 분석하여 상품 추천하기¶
이번 절에서는 구매 데이터 분석에 기반한 온라인 스토어 상품 추천 시뮬레이션 예제에 대해 알아보자. 예제에서 피처 엔지니어링, 그리고 행렬 완성 기반 점수 예측 방법을 이용하여 상품 추천 시뮬레이션을 수행한다. 분석에 사용할 데이터는 영국의 한 선물 판매 온라인 스토어에서 발생한 거래 데이터로, 주 고객은 선물 도매상이다.
step.1 탐색적 분석: UK Retail 데이터 분석하기¶
예제에서 사용할 UK Retail 데이터셋은 다음과 같은 피처로 구성되어 있다.
- InvoiceNo : 거래 고유 번호
- StockCode : 상품 고유 번호
- Description : 상품명
- Quantity : 거래 수량
- InvoiceDate : 거래 일시
- UnitPrice : 상품 단가
- CustomerID : 구매자 고유 번호
- Country : 구매 국가
아래 코드를 실행해 보면 약 54만 개 정도의 데이터가 존재하고 그 중 14만 개의 데이터는 구매자 정보가 결측값인 것을 알 수 있다.
# -*- coding: utf-8 -*-
%matplotlib inline
import pandas as pd
import numpy as np
import matplotlib.pyplot as plt
import warnings
warnings.filterwarnings("ignore")
- 데이터셋 살펴보기
# 영국 온라인 스토어 도매 거래 데이터
df = pd.read_csv('/home/jaeyoon89/python-data-analysis/data/online_retail.csv', dtype={'CustomerID':str,'InvoicedID':str},encoding="ISO-8859-1")
df['InvoiceDate'] = pd.to_datetime(df['InvoiceDate'], format="%m/%d/%Y %H:%M")
print(df.info())
df.head()
<class 'pandas.core.frame.DataFrame'> RangeIndex: 541909 entries, 0 to 541908 Data columns (total 8 columns): # Column Non-Null Count Dtype --- ------ -------------- ----- 0 InvoiceNo 541909 non-null object 1 StockCode 541909 non-null object 2 Description 540455 non-null object 3 Quantity 541909 non-null int64 4 InvoiceDate 541909 non-null datetime64[ns] 5 UnitPrice 541909 non-null float64 6 CustomerID 406829 non-null object 7 Country 541909 non-null object dtypes: datetime64[ns](1), float64(1), int64(1), object(5) memory usage: 33.1+ MB None
InvoiceNo | StockCode | Description | Quantity | InvoiceDate | UnitPrice | CustomerID | Country | |
---|---|---|---|---|---|---|---|---|
0 | 536365 | 85123A | WHITE HANGING HEART T-LIGHT HOLDER | 6 | 2010-12-01 08:26:00 | 2.55 | 17850 | United Kingdom |
1 | 536365 | 71053 | WHITE METAL LANTERN | 6 | 2010-12-01 08:26:00 | 3.39 | 17850 | United Kingdom |
2 | 536365 | 84406B | CREAM CUPID HEARTS COAT HANGER | 8 | 2010-12-01 08:26:00 | 2.75 | 17850 | United Kingdom |
3 | 536365 | 84029G | KNITTED UNION FLAG HOT WATER BOTTLE | 6 | 2010-12-01 08:26:00 | 3.39 | 17850 | United Kingdom |
4 | 536365 | 84029E | RED WOOLLY HOTTIE WHITE HEART. | 6 | 2010-12-01 08:26:00 | 3.39 | 17850 | United Kingdom |
본격적인 탐색적 데이터 분석에 앞서, 데이터에서 예외적인 상황을 필터링하여 이상치를 제거해야 한다. 가장 먼저 결측 데이터를 제거하자. 다음 코드 결과는 유저정보가 없는 13만 5천여 개의 데이터, 상품 상세정보가 없는 1500여 개의 데이터를 제거한 것이다.
- 결측 데이터 제거하기
df.isnull().sum()
InvoiceNo 0 StockCode 0 Description 1454 Quantity 0 InvoiceDate 0 UnitPrice 0 CustomerID 135080 Country 0 dtype: int64
df = df.dropna()
print(df.shape)
(406829, 8)
다음은 데이터가 일반적이지 않은 경우를 탐색하고 이를 제거하자. 이번에는 상품 수량 데이터가 이상한 경우를 탐색해보자. 아래의 코드는 상품수량이 0 이하인 경우 해당 값을 데이터 프레임에서 제거하는 과정이다.
- 탐색 데이터의 조건 필터링 : 상품 수량이 0 이하인 경우
# 상품 수량이 음수인 경우를 제거한다.
print(df[df['Quantity']<=0].shape[0])
df = df[df['Quantity']>0]
8905
이번엔 상품 가격이 0 이하인 경우를 탐색하자. 역시 같은 방법으로 제거해보자.
- 탐색 데이터의 조건 필터링 : 상품가격이 0 이하인 경우
# 상품 가격이 0 이하인 경우를 제거한다.
print(df[df['UnitPrice']<=0].shape[0])
df = df[df['UnitPrice']>0]
40
마지막으로 상품 코드가 이상한 경우를 탐색하고 제거하자. 데이터 내의 StockCode를 관찰해보면 대부분의 상품 코드가 번호로 이루어져 있는 것을 알 수 있다. 따라서 상품 코드가 번호가 아닌 경우는 예외적인 상황일 것이다.
- 탐색 데이터의 조건 필터링 : 상품 코드가 일반적이지 않은 경우
# 상품 코드가 일반적이지 않은 경우를 탐색한다.
df['ContainDigit'] = df['StockCode'].apply(lambda x: any(c.isdigit() for c in x))
print(df[df['ContainDigit'] == False].shape[0])
df[df['ContainDigit'] == False].head()
1414
InvoiceNo | StockCode | Description | Quantity | InvoiceDate | UnitPrice | CustomerID | Country | ContainDigit | |
---|---|---|---|---|---|---|---|---|---|
45 | 536370 | POST | POSTAGE | 3 | 2010-12-01 08:45:00 | 18.00 | 12583 | France | False |
386 | 536403 | POST | POSTAGE | 1 | 2010-12-01 11:27:00 | 15.00 | 12791 | Netherlands | False |
1123 | 536527 | POST | POSTAGE | 1 | 2010-12-01 13:04:00 | 18.00 | 12662 | Germany | False |
2239 | 536569 | M | Manual | 1 | 2010-12-01 15:35:00 | 1.25 | 16274 | United Kingdom | False |
2250 | 536569 | M | Manual | 1 | 2010-12-01 15:35:00 | 18.95 | 16274 | United Kingdom | False |
# 상품 코드가 일반적이지 않은 경우를 제거한다.
df = df[df['ContainDigit'] == True]
본격적으로 탐색적 분석을 수행할 차례이다. 우리는 어떤 방향성을 가지고 탐색적 분석을 해야할까? 그러려면 우리가 온라인 스토어의 운영자 입장이 되어 생각을 해보자. 연말에 방문한 유저들에게 상품을 추천해준다는 것은 유저-상품 간의 구매 확률을 예측해보는 시뮬레이션이라고 할 수 있다. 이러한 예측 시뮬레이션은 아래와 같은 분석 과정이 필요하다.
- 연말 이전까지의 데이터를 유저-상품 간의 구매를 예측하는 모델의 학습 데이터셋으로 사용한다.
- 실제 연말에 구매한 유저-상품 간의 정보를 테스트 데이터셋으로 사용한다.
- 모델이 예측한 유저-상품 간의 구매 정보와 실제 구매 정보(테스트 데이터셋)을 비교하여 추천이 잘 되었는지 평가한다.
- 데이터의 기간 탐색하기
# 거래 데이터에서 가장 오래된 데이터와 가장 최신의 데이터를 탐색한다.
df['date'] = df['InvoiceDate'].dt.date
print(df['date'].min())
print(df['date'].max())
2010-12-01 2011-12-09
다음으로 일자별 거래량을 탐색하자. 코드에서는 일자를 나타내는 date 피처를 그룹의 기준으로 하여, 일자별 Quantity의 합계를 계산하였다.
- 일자별 거래 수량 탐색하기
# 일자별 총 거래 수량을 탐색하자.
date_quantity_series = df.groupby('date')['Quantity'].sum()
date_quantity_series.plot()
<AxesSubplot:xlabel='date'>
다음은 일자별 거래 횟수를 탐색하자. 아래의 코드도 date 피처를 그룹의 기준으로 하였고, nunique() 함수를 InvoiceNo 피처에 적용하여 일자별로 발생한 거래 횟수를 계산한다.
- 일자별 거래 횟수 탐색하기
# 일자별 총 거래 횟수를 탐색한다.
date_transaction_series = df.groupby('date')['InvoiceNo'].nunique()
date_transaction_series.plot()
<AxesSubplot:xlabel='date'>
마지막으로 일자별 거래 상품 개수를 탐색하자. 아래 결과를 보면 지금까지의 그래프중 가장 가파른 상승세를 보이고 있다.
- 일자별 거래 상품 개수 탐색하기
# 일자별 거래된 상품의 unique한 개수, 즉 상품 거래 다양성을 탐색한다.
date_unique_item_series = df.groupby('date')['StockCode'].nunique()
date_unique_item_series.plot()
<AxesSubplot:xlabel='date'>
이번엔 전체 데이터에서 등장한 유저들의 구매 패턴을 탐색해보자.
- 전체 유저의 수 탐색하기
# 총 유저의 수를 계산하여 출력한다.
print(len(df['CustomerID'].unique()))
4334
총 4334명의 유저를 대상으로 각각의 거래 횟수를 탐색하자. 아래 코드는 CustomerID를 그룹으로 하여 unique한 InvoiceNo를 계산한 것이고, 이 결과에 describe() 함수를 적용하여 유저별 거래 횟수에 대한 요약 통계 정보를 출력하였다.
- 유저별 거래 횟수 탐색하기
# 유저별 거래 횟수를 탐색한다.
customer_unique_transaction_series = df.groupby('CustomerID')['InvoiceNo'].nunique()
customer_unique_transaction_series.describe()
count 4334.000000 mean 4.246654 std 7.642535 min 1.000000 25% 1.000000 50% 2.000000 75% 5.000000 max 206.000000 Name: InvoiceNo, dtype: float64
그리고 이를 상자 그림으로 살펴본 결과는 다음과 같다.
- 유저별 거래횟수 시각화하기
# 상자 그림 시각화로 살펴보자.
plt.boxplot(customer_unique_transaction_series.values)
plt.show()
다음으로 유저별로 구매한 상품은 몇 종류나 되는지를 탐색해보자. 아래의 코드는 CustomerID그룹에 unique한 StockCode를 계산하여 describe() 함수를 적용한 것이다.
- 유저별 상품 구매 종류 탐색하기
# 유저별 아이템 구매 종류 개수를 탐색하자.
customer_unique_item_series = df.groupby('CustomerID')['StockCode'].nunique()
customer_unique_item_series.describe()
count 4334.000000 mean 61.432856 std 85.312937 min 1.000000 25% 16.000000 50% 35.000000 75% 77.000000 max 1786.000000 Name: StockCode, dtype: float64
마찬가지로 상자그림으로 살펴보자.
- 유저별 상품 구매 종류 시각화하기
# 상자 그림 시각화로 살펴보자.
plt.boxplot(customer_unique_item_series.values)
plt.show()
이제 특정 시점을 기준으로 데이터를 분리하여 구매의 패턴을 분석해보자. 중점적으로 살펴볼 내용은 두 데이터에서 동일하게 등장하는 유저-상품 단위의 구매 데이터, 즉 재구매 여부이다. 또한 신규 구매가 얼마나 일어났는지 역시 중요하게 살펴볼 내용이다. 먼저 11월 1일을 연말의 기준으로 삼아 두 개의 데이터로 분리한다.
- 일자를 기준으로 데이터 분리하기
import datetime
# 2011년 11월을 기준으로 하여 기준 이전과 이후로 데이터를 분리한다.
df_year_round = df[df['date'] < datetime.date(2011, 11, 1)]
df_year_end = df[df['date'] >= datetime.date(2011, 11, 1)]
print(df_year_round.shape)
print(df_year_end.shape)
(314902, 10) (81568, 10)
분리된 데이터에서 재구매, 신규 구매 등이 어떻게 일어났는지를 분석해보자. 먼저 해야 하는 것은 11월 이전 데이터셋에서 유저별로 구매했던 상품의 리스트를 추출하는 것이다.
- 11월 이전 유저별로 구매했던 상품의 집합 추출하기
# 11월 이전 데이터에서 구매했던 상품의 set을 추출한다.
customer_item_round_set = df_year_round.groupby('CustomerID')['StockCode'].apply(set)
print(customer_item_round_set)
CustomerID 12346 {23166} 12347 {47567B, 23146, 84992, 23421, 22195, 23422, 22... 12348 {21983, 21211, 21725, 22951, 21982, 23076, 849... 12350 {84086C, 20652, 21832, 21915, 20615, 21864, 21... 12352 {21770, 22722, 22801, 22624, 84510A, 21914, 21... ... 18280 {82484, 22180, 22727, 22495, 22467, 22358, 226... 18281 {23209, 22716, 23008, 22028, 22037, 22467, 23007} 18282 {21108, 21109, 22089, 23295, 22424, 21270, 23187} 18283 {22328, 22149, 22384, 22653, 22960, 21068, 219... 18287 {85040A, 22754, 22865, 22600, 21824, 23274, 22... Name: StockCode, Length: 3970, dtype: object
다음은 유저-상품 단위의 딕셔너리를 정의하자. 이 딕셔너리는 유저가 상품을 11월 이전에 구매했는지 혹은 11월 이후에 구매했는지를 기록하기 위한 것이다.
- 유저별 구매 사전 구축하기
# 11월 이전에 구매했는지 혹은 이후에 구매했는지를 유저별로 기록하기 위한 사전을 정의한다.
customer_item_dict = {}
# 11월 이전에 구매한 상품은 'old'라고 표기한다.
for customer_id, stocks in customer_item_round_set.items():
customer_item_dict[customer_id] = {}
for stock_code in stocks:
customer_item_dict[customer_id][stock_code] = 'old'
print(str(customer_item_dict)[:100] + "...")
{'12346': {'23166': 'old'}, '12347': {'47567B': 'old', '23146': 'old', '84992': 'old', '23421': 'old...
위와 동일한 방식으로 11월 이후 데이터에서 유저별로 구매한 상품의 집합을 추출하자.
- 11월 이후 유저별로 구매했던 상품의 집합 추출하기
# 11월 이후 데이터에서 구매하는 상품의 집합을 추출한다.
customer_item_end_set = df_year_end.groupby('CustomerID')['StockCode'].apply(set)
print(customer_item_end_set)
CustomerID 12347 {21265, 23552, 23497, 23271, 23084, 84625A, 21... 12349 {47504H, 22430, 21086, 21533, 22601, 48184, 22... 12352 {22668, 22982, 22627, 22624, 23089, 21669, 233... 12356 {22423, 21843} 12357 {22984, 15056BL, 23485, 22306, 35598D, 22357, ... ... 18272 {72799E, 22666, 22960, 22076, 72799C, 22075, 8... 18273 {79302M} 18274 {22423, 21108, 22720, 21231, 22851, 23245, 845... 18282 {22423, 22818, 22699, 23174, 23175} 18283 {22384, 22732, 21889, 23377, 22614, 22572, 223... Name: StockCode, Length: 1904, dtype: object
11월 이후에 구매한 유저별 상품의 집합을 이용하여 앞서 정의했던 유저-상품 구매 상태 딕셔너리를 업데이트한다.
- 유저별 구매 사전 업데이트하기
# 11월 이전에만 구매한 상품은 'old', 이후에만 구매한 상품은 'new', 모두 구매한 상품은 'both'라고 표기한다.
for customer_id, stocks in customer_item_end_set.items():
# 11월 이전 구매기록이 있는 유저인지를 체크한다.
if customer_id in customer_item_dict:
for stock_code in stocks:
# 구매한 적 있는 상품인지를 체크한 뒤, 상태를 표기한다.
if stock_code in customer_item_dict[customer_id]:
customer_item_dict[customer_id][stock_code] = 'both'
else:
customer_item_dict[customer_id][stock_code] = 'new'
# 11월 이전 구매기록이 없는 유저라면 모두 'new'로 표기한다.
else:
customer_item_dict[customer_id] = {}
for stock_code in stocks:
customer_item_dict[customer_id][stock_code] = 'new'
print(str(customer_item_dict)[:100] + "...")
{'12346': {'23166': 'old'}, '12347': {'47567B': 'old', '23146': 'old', '84992': 'old', '23421': 'old...
다음으로 구축 완료된 딕셔너리를 조금 더 편하게 분석하기 위해 데이터 프레임의 형태로 다시 정리하자.
- 구매 사전을 데이터 프레임으로 정리하기
# 'old', 'new', 'both'를 유저별로 탐색하여 데이터 프레임을 생성한다.
columns = ['CustomerID', 'old', 'new', 'both']
df_order_info = pd.DataFrame(columns=columns)
# 데이터 프레임을 생성하는 과정이다.
for customer_id in customer_item_dict:
old = 0
new = 0
both = 0
# 딕셔너리의 상품 상태(old, new, both)를 체크하여 데이터 프레임에 append 할 수 있는 형태로 처리한다.
for stock_code in customer_item_dict[customer_id]:
status = customer_item_dict[customer_id][stock_code]
if status == 'old':
old += 1
elif status == 'new':
new += 1
else:
both += 1
# df_order_info에 데이터를 append한다.
row = [customer_id, old, new, both]
series = pd.Series(row, index=columns)
df_order_info = df_order_info.append(series, ignore_index=True)
df_order_info.head()
CustomerID | old | new | both | |
---|---|---|---|---|
0 | 12346 | 1 | 0 | 0 |
1 | 12347 | 92 | 3 | 8 |
2 | 12348 | 21 | 0 | 0 |
3 | 12350 | 16 | 0 | 0 |
4 | 12352 | 43 | 12 | 2 |
이렇게 정리된 데이터 프레임을 활용해 재구매와 신규 구매가 어떤 패턴으로 발생하였는지 탐색해보자.
- 재구매, 신규 구매 유저 분석하기
# 데이터 프레임에서 전체 유저 수를 출력한다.
print(df_order_info.shape[0])
4334
# 데이터 프레임에서 old가 1 이상이면서, new가 1 이상인 유저수를 출력한다.
# 11월 이후에 기존에 구매한 적 없는 새로운 상품을 구매한 유저를 의미한다.
print(df_order_info[(df_order_info['old']>0) & (df_order_info['new']>0)].shape[0])
1446
# 데이터 프레임에서 both가 1 이상인 유저 수를 출력한다.
# 재구매한 상품이 있는 유저의 수를 의미한다.
print(df_order_info[df_order_info['both'] > 0].shape[0])
1426
신규 구매한 상품이 있는 유저들은 얼마나 많은 종류의 신규 상품을 구매했는지를 탐색한다.
- 신규 구매 상품 종류 탐색하기
# 만약 새로운 상품을 구매한다면 얼마나 많은 종류의 새로운 상품을 구매하는지 탐색한다.
print(df_order_info['new'].value_counts()[1:].describe())
count 132.000000 mean 13.734848 std 19.130672 min 1.000000 25% 1.000000 50% 5.000000 75% 16.000000 max 81.000000 Name: new, dtype: float64
step.2 예측 분석: SVD를 활용한 상품 구매 예측하기¶
지금까지 탐색한 내용을 토대로 상품 추천 시뮬레이션을 준비해보자. 앞서 설명한 대로 상품 추천 시뮬레이션이라는 것은 과거의 학습 데이터셋을 이용하여 미래의 유저-상품 구매를 예측하는 것이다. 우선 SVD 예측 모델 학습을 진행하기에 앞서 학습 데이터인 11월 이전의 데이터에서 추천 대상이 되는 유저와 상품은 얼마나 되는지를 탐색해보자.
- 추천 대상인 유저와 상품 출력하기
# 추천 대상 데이터에 포함되는 유저와 상품의 개수를 출력한다.
print(len(df_year_round['CustomerID'].unique()))
print(len(df_year_round['StockCode'].unique()))
3970 3608
SVD 모델을 학습하면서 앞장에서 배웠던 내용과 다른점은 유저-아이템의 'Rating'에 해당하는 선호도 점수를 가지지 않고 있다는 것이다. 이 문제를 해결하기 위해선 바로 피처 엔지니어링을 통해 이 점수를 만들어내야 한다. 다음 코드는 유저-상품 간의 구매 횟수를 계산하여 U-I-R 데이터로 활용할 데이터 프레임을 생성하는 과정이다.
- SVD 모델에 사용할 Rating 탐색하기
# Rating 데이터를 생성하기 위한 탐색: 유저-상품간 구매 횟수를 탐색한다.
uir_df = df_year_round.groupby(['CustomerID', 'StockCode'])['InvoiceNo'].nunique().reset_index()
uir_df.head()
CustomerID | StockCode | InvoiceNo | |
---|---|---|---|
0 | 12346 | 23166 | 1 |
1 | 12347 | 16008 | 1 |
2 | 12347 | 17021 | 1 |
3 | 12347 | 20665 | 1 |
4 | 12347 | 20719 | 3 |
이렇게 Rating이라고 가정한 유저-상품 간의 구매 횟수가 어떻게 분포되어 있는지를 그래프로 탐색해 본 결과는 아래와 같다.
# Rating(InvoiceNo) 피처의 분포를 탐색한다.
uir_df['InvoiceNo'].hist(bins=20, grid=False)
<AxesSubplot:>
이러한 상황에서 적용할 수 있는 피처 엔지니어링 기법으로 로그를 통한 피처 정규화 방법이 있다. 이 방법의 목적은 위 그래프에 나타난 것처럼 데이터의 왜도(한쪽으로 긴 꼬리를 가진 형태의 비대칭적인 분포 정도) 가 높은 경우에 '데이터 사이의 편차를 줄여 왜도를 감소시키는 것' 에 있다.
- Log Normalization 적용하기
# Rating(InvoiceNo) 피처를 log normalization 해준 뒤, 다시 분포를 탐색한다.
uir_df['InvoiceNo'].apply(lambda x: np.log10(x)+1).hist(bins=20, grid=False)
<AxesSubplot:>
로그를 통한 정규화 적용 후, 다시 피처 스케일링을 적용하여 1 ~ 5 사이의 값으로 변환한다. 아래의 코드는 변환 이후의 분포를 다시 그래프로 출력한 것이다. 코드에서는 최대-최소 스케일링 방법을 적용하였다.
- 피처 스케일링 적용하기
# 1~5 사이의 점수로 변환한다.
uir_df['Rating'] = uir_df['InvoiceNo'].apply(lambda x: np.log10(x)+1)
uir_df['Rating'] = ((uir_df['Rating']- uir_df['Rating'].min()) / (uir_df['Rating'].max() - uir_df['Rating'].min()) * 4) + 1
uir_df['Rating'].hist(bins=20, grid=False)
<AxesSubplot:>
유저-상품 간의 Rating 점수를 정의하여 우리에게 필요했던 U-I-R 매트릭스 데이터가 완성 되었다. 이제 SVD 모델을 학습해 보자.
- SVD 모델 학습을 위한 데이터셋 생성하기
# SVD 모델 학습을 위한 데이터셋을 생성한다.
uir_df = uir_df[['CustomerID','StockCode','Rating']]
uir_df.head()
CustomerID | StockCode | Rating | |
---|---|---|---|
0 | 12346 | 23166 | 1.000000 |
1 | 12347 | 16008 | 1.000000 |
2 | 12347 | 17021 | 1.000000 |
3 | 12347 | 20665 | 1.000000 |
4 | 12347 | 20719 | 2.048881 |
이렇게 생성된 데이터셋으로 SVD 모델을 학습하자. 모델의 대략적인 성능을 알아보기 위해 11월 이전 데이터로 생성한 학습 데이터인 uir_df를 또 다시 학습 데이터와 테스트 데이터셋으로 분리하여 모델을 학습하고 평가한다.
- SVD 모델 성능 테스트하기
import time
from surprise import SVD, Dataset, Reader, accuracy
from surprise.model_selection import train_test_split
# SVD 라이브러리를 사용하기 위한 학습 데이터를 생성한다. 대략적인 성능을 알아보기 위해 학습 데이터와 테스트 데이터를 8:2로 분할한다.
reader = Reader(rating_scale=(1, 5))
data = Dataset.load_from_df(uir_df[['CustomerID', 'StockCode', 'Rating']], reader)
train_data, test_data = train_test_split(data, test_size=0.2)
# SVD 모델을 학습한다.
train_start = time.time()
model = SVD(n_factors=8,
lr_all=0.005,
reg_all=0.02,
n_epochs=200)
model.fit(train_data)
train_end = time.time()
print("training time of model : %.2f seconds" % (train_end - train_start))
predictions = model.test(test_data)
# 테스트 데이터의 RMSE를 출력하여 모델의 성능을 평가한다.
print("RMSE of test dataset in SVD model:")
accuracy.rmse(predictions)
training time of model : 21.49 seconds RMSE of test dataset in SVD model: RMSE: 0.3373
0.33730303362362735
모델의 대략적인 성능을 알아보았으니 이제 11월 데이터를 모두 학습 데이터로만 사용하여 모델을 학습한다.
- 전체 학습 데이터로 SVD 모델 학습하기
# SVD 라이브러리를 사용하기 위한 학습 데이터를 생성한다. 11월 이전 전체를 full trainset으로 활용한다.
reader = Reader(rating_scale=(1,5))
data = Dataset.load_from_df(uir_df[['CustomerID','StockCode','Rating']], reader)
train_data = data.build_full_trainset()
# SVD 모델을 학습한다.
train_start = time.time()
model = SVD(n_factors=8,
lr_all=0.005,
reg_all=0.02,
n_epochs=200)
model.fit(train_data)
train_end = time.time()
print("training time of model: %.2f seconds" % (train_end - train_start))
training time of model: 23.87 seconds
step.3 예측 평가: 상품 추천 시뮬레이션하기¶
이번 단계에서는 앞서 학습한 SVD 모델을 활용해 상품 추천 시뮬레이션을 수행해보자. 추천의 대상이 되는 유저는 11월 이전 데이터에 등장한 모든 유저를 대상으로 한다. 반면, 유저들에게 추천의 대상이 되는 상품은 아래의 3가지로 분류할 수 있다.
- 이전에 구매한 적 없던 상품 추천 : 신규 구매를 타겟으로 하는 추천
- 이전에 구매했던 상품 추천 : 재구매를 타겟으로 하는 추천
- 모든 상품을 대상으로 상품 추천 : 모든 유저-상품의 점수를 고려하여 추천
- 첫 번째 추천 대상의 유저-상품 점수 추출하기
# 이전에 구매하지 않았던 상품을 예측의 대상으로 선정한다.
test_data = train_data.build_anti_testset()
target_user_predictions = model.test(test_data)
# 구매 예측 결과를 딕셔너리 형태로 변환한다.
new_order_prediction_dict = {}
for customer_id, stock_code, _, predicted_rating, _ in target_user_predictions:
if customer_id in new_order_prediction_dict:
if stock_code in new_order_prediction_dict[customer_id]:
pass
else:
new_order_prediction_dict[customer_id][stock_code] = predicted_rating
else:
new_order_prediction_dict[customer_id] = {}
new_order_prediction_dict[customer_id][stock_code] = predicted_rating
print(str(new_order_prediction_dict)[:300] + "...")
{'12346': {'16008': 1.0025127099786768, '17021': 1.1760169333017347, '20665': 1.0003706315176795, '20719': 1.2594873292681599, '20780': 1, '20782': 1.1349496493887574, '20966': 1.0284717853462468, '21035': 1.1166930324150979, '21041': 1.102268131359537, '21064': 1.0763187667518186, '21154': 1.130583...
마찬가지의 방법으로 두 번째 추천 대상인 데이터에 등장한 모든 유저-이전에 구매했던 상품 간의 예측 점수를 딕셔너리 형태로 추출하는 과정을 수행하자. 이번에는 build_anti_testset() 함수대신 build_testset() 함수를 사용하여 이전에 구매했었던 상품을 대상으로 test_data를 추출한다.
- 두 번째 추천 대상의 유저-상품 점수 추출하기
# 이전에 구매했었던 상품을 예측의 대상으로 선정한다.
test_data = train_data.build_testset()
target_user_predictions = model.test(test_data)
# 구매 예측 결과를 딕셔너리 형태로 변환한다.
reorder_prediction_dict = {}
for customer_id, stock_code, _, predicted_rating, _ in target_user_predictions:
if customer_id in reorder_prediction_dict:
if stock_code in reorder_prediction_dict[customer_id]:
pass
else:
reorder_prediction_dict[customer_id][stock_code] = predicted_rating
else:
reorder_prediction_dict[customer_id] = {}
reorder_prediction_dict[customer_id][stock_code] = predicted_rating
print(str(reorder_prediction_dict)[:300] + "...")
{'12346': {'23166': 1.0122048478213461}, '12347': {'16008': 1.285509992669434, '17021': 1.4039490567043034, '20665': 1.094534385550671, '20719': 1.7181798796897685, '20780': 1.1183456103591423, '20782': 1.3550617174804296, '20966': 1.2670250033610035, '21035': 1.3387673825725332, '21041': 1.40224244...
세 번째 추천 대상은 위에서 생선한 두 개의 딕셔너리를 하나의 딕셔너리로 통합하는 과정으로 생성 할 수 있다. 다음 코드로 모든 유저와 모든 상품 간의 예측 점수를 딕셔너리 형태로 저장한다.
- 세 번째 추천 대상의 유저-상품 점수 추출하기
# 두 딕셔너리를 하나로 통합한다.
total_prediction_dict = {}
# new_order_prediction_dict 정보를 새로운 딕셔너리에 저장한다.
for customer_id in new_order_prediction_dict:
if customer_id not in total_prediction_dict:
total_prediction_dict[customer_id] = {}
for stock_code, predicted_rating in new_order_prediction_dict[customer_id].items():
if stock_code not in total_prediction_dict[customer_id]:
total_prediction_dict[customer_id][stock_code] = predicted_rating
# reorder_prediction_dict 정보를 새로운 딕셔너리에 저장한다.
for customer_id in reorder_prediction_dict:
if customer_id not in total_prediction_dict:
total_prediction_dict[customer_id] = {}
for stock_code, predicted_rating in reorder_prediction_dict[customer_id].items():
if stock_code not in total_prediction_dict[customer_id]:
total_prediction_dict[customer_id][stock_code] = predicted_rating
print(str(total_prediction_dict)[:300] + "...")
{'12346': {'16008': 1.0025127099786768, '17021': 1.1760169333017347, '20665': 1.0003706315176795, '20719': 1.2594873292681599, '20780': 1, '20782': 1.1349496493887574, '20966': 1.0284717853462468, '21035': 1.1166930324150979, '21041': 1.102268131359537, '21064': 1.0763187667518186, '21154': 1.130583...
앞의 단계들을 거쳐 우리는 세 가지 상품 추천의 시뮬레이션 결과를 각각의 딕셔너리 형태로 갖게 되었다. 그렇다면 이제 시뮬레이션 결과가 실제 구매와 얼마나 유사한지 평가해보자.
- 시뮬레이션을 테스트할 데이터 프레임 생성하기
# 11월 이후의 데이터를 테스트 데이터셋으로 사용하기 위한 데이터 프레임을 생성한다.
simulation_test_df = df_year_end.groupby('CustomerID')['StockCode'].apply(set).reset_index()
simulation_test_df.columns = ['CustomerID','RealOrdered']
simulation_test_df.head()
CustomerID | RealOrdered | |
---|---|---|
0 | 12347 | {21265, 23552, 23497, 23271, 23084, 84625A, 21... |
1 | 12349 | {47504H, 22430, 21086, 21533, 22601, 48184, 22... |
2 | 12352 | {22668, 22982, 22627, 22624, 23089, 21669, 233... |
3 | 12356 | {22423, 21843} |
4 | 12357 | {22984, 15056BL, 23485, 22306, 35598D, 22357, ... |
시뮬레이션 테스트용 데이터 프레임에 3개 딕셔너리의 시뮬레이션 결과를 추가한다. 이를 위해 아래 코드와 같이 add_predicted_stock_set()이라는 함수를 정의하자.
- 시뮬레이션 결과 추가하기
# 이 데이터 프레임에 상품 추천 시뮬레이션 결과를 추가하기 위한 함수를 정의한다.
def add_predicted_stock_set(customer_id, prediction_dict):
if customer_id in prediction_dict:
predicted_stock_dict = prediction_dict[customer_id]
# 예측된 상품의 Rating이 높은 순으로 정렬한다.
sorted_stocks = sorted(predicted_stock_dict, key=lambda x: predicted_stock_dict[x], reverse=True)
return sorted_stocks
else:
return None
# 상품 추천 시뮬레이션 결과를 추가한다.
simulation_test_df['PredictedOrder(New)'] = simulation_test_df['CustomerID']. \
apply(lambda x: add_predicted_stock_set(x, new_order_prediction_dict))
simulation_test_df['PredictedOrder(Reorder)'] = simulation_test_df['CustomerID']. \
apply(lambda x: add_predicted_stock_set(x, reorder_prediction_dict))
simulation_test_df['PredictedOrder(Total)'] = simulation_test_df['CustomerID']. \
apply(lambda x: add_predicted_stock_set(x, total_prediction_dict))
simulation_test_df.head()
CustomerID | RealOrdered | PredictedOrder(New) | PredictedOrder(Reorder) | PredictedOrder(Total) | |
---|---|---|---|---|---|
0 | 12347 | {21265, 23552, 23497, 23271, 23084, 84625A, 21... | [22197, 22659, 37448, 23199, 23301, 22616, 223... | [22726, 22728, 22727, 22729, 22371, 22725, 217... | [22726, 22197, 22659, 37448, 22728, 22727, 231... |
1 | 12349 | {47504H, 22430, 21086, 21533, 22601, 48184, 22... | None | None | None |
2 | 12352 | {22668, 22982, 22627, 22624, 23089, 21669, 233... | [84086B, 90119, 85123A, 90035A, 85131B, 82582,... | [22779, 22780, 21914, 21756, 21754, 22413, 374... | [84086B, 90119, 85123A, 90035A, 85131B, 82582,... |
3 | 12356 | {22423, 21843} | [84086B, 85131B, 90119, C2, 90042A, 20914, 850... | [22423, 21843, 22649, 22699, 21086, 37450, 849... | [84086B, 85131B, 90119, C2, 90042A, 20914, 850... |
4 | 12357 | {22984, 15056BL, 23485, 22306, 35598D, 22357, ... | None | None | None |
총 3개의 상품 추천 시뮬레이션 결과가 추가되었다.
이제 테스트 데이터셋을 완성하였으니 추천 시뮬레이션이 실제 구매와 얼마나 비슷하게 예측되었는지 평가해보자. 우리가 사용할 방식은 다음과 같다.
- 1.유저별로 예측된 상품의 점수 순으로 상위k개의 상품을 추천 대상으로 정의한다.
- 2.추천한 k개의 상품 중, 실제 구매로 얼마만큼 이어졌는지 평가한다.
다음 코드의 calculate_recall() 함수는 이 과정을 코드로 정의한 것이다. real_order 파라미터는 실제 구매한 상품의 리스트이고, predicted_order 파라미터는 예측 점수순으로 정렬된 상품의 리스트이다. 그리고 k 파라미터는 추천할 개수를 의미한다.
- 상품 추천 평가 기준 정의하기
# 구매 예측의 상위 k개의 recall(재현율)을 평가 기준으로 정의한다.
def calculate_recall(real_order, predicted_order, k):
# 만약 추천 대상 상품이 없다면, 11월 이후에 상품을 처음 구매하는 유저이다.
if predicted_order is None:
return None
# SVD 모델에서 현재 유저의 Rating이 높은 상위 k개의 상품을 "구매 할 것으로 예측"한다.
predicted = predicted_order[:k]
true_positive = 0
for stock_code in predicted:
if stock_code in real_order:
true_positive += 1
# 예측한 상품 중, 실제로 유저가 구매한 상품의 비율(recall)을 계산한다.
recall = true_positive / len(predicted)
return recall
위에서 정의한 함수를 apply()로 적용하여 3개의 시뮬레이션 평가 결과를 저장한다. 실행 결과 생성되는 피처는 점수순으로 k개의 상품을 추천해 주었을 때의 재현도(recall) 점수를 계산한 것이다.
- 상품 추천 평가하기
# 시뮬레이션 대상 유저에게 상품을 추천해준 결과를 평가합니다.
simulation_test_df['top_k_recall(Reorder)'] = simulation_test_df. \
apply(lambda x: calculate_recall(x['RealOrdered'],
x['PredictedOrder(Reorder)'],
5), axis=1)
simulation_test_df['top_k_recall(New)'] = simulation_test_df. \
apply(lambda x: calculate_recall(x['RealOrdered'],
x['PredictedOrder(New)'],
5), axis=1)
simulation_test_df['top_k_recall(Total)'] = simulation_test_df. \
apply(lambda x: calculate_recall(x['RealOrdered'],
x['PredictedOrder(Total)'],
5), axis=1)
- 평가 결과 출력하기
# 평가 결과를 유저 평균으로 살펴보자.
print(simulation_test_df['top_k_recall(Reorder)'].mean())
print(simulation_test_df['top_k_recall(New)'].mean())
print(simulation_test_df['top_k_recall(Total)'].mean())
0.30840909090909097 0.005844155844155844 0.06805194805194804
이를 통해 우리는 재구매할만한 상품을 추천해주는 것이 새로운 상품을 추천해주는 것보다 더 좋은 결과를 낼 것이라고 예상할 수 있다. 다음으로 추천 시뮬레이션 각각의 세부 결과를 살표보자.
- 재구매 상품 추천의 상세 결과
# 평가 결과를 점수 기준으로 살펴본다.
simulation_test_df['top_k_recall(Reorder)'].value_counts()
0.000000 474 0.200000 412 0.400000 248 0.600000 205 0.800000 102 1.000000 80 0.500000 7 0.250000 6 0.666667 4 0.333333 1 0.750000 1 Name: top_k_recall(Reorder), dtype: int64
- 신규 상품 추천의 상세 결과
# 평가 결과를 점수 기준으로 살펴보자.
simulation_test_df['top_k_recall(New)'].value_counts()
0.0 1498 0.2 39 0.4 3 Name: top_k_recall(New), dtype: int64
- 전체 상품 추천의 상세 결과
# 평가 결과를 점수 기준으로 살펴보자.
simulation_test_df['top_k_recall(Total)'].value_counts()
0.0 1236 0.2 178 0.4 60 0.6 45 0.8 14 1.0 7 Name: top_k_recall(Total), dtype: int64
3개의 시뮬레이션 결과 재구매할만한 상품을 추천해 주는 것이 가장 좋은 시뮬레이션인 것으로 평가 되었다.
- 시뮬레이션 최종 결과 정리
# 추천 시뮬레이션 결과를 살펴보자.
k = 5
result_df = simulation_test_df[simulation_test_df['PredictedOrder(Reorder)'].notnull()]
result_df['PredictedOrder(Reorder)'] = result_df['PredictedOrder(Reorder)'].\
apply(lambda x: x[:k])
result_df = result_df[['CustomerID', 'RealOrdered',
'PredictedOrder(Reorder)', 'top_k_recall(Reorder)']]
result_df.columns = [['구매자ID', '실제주문', '5개추천결과', 'Top5추천_주문재현도']]
result_df.sample(5).head()
구매자ID | 실제주문 | 5개추천결과 | Top5추천_주문재현도 | |
---|---|---|---|---|
1726 | 17719 | {23167, 20983, 22665, 22720, 23341, 22077, 227... | [22726, 22727, 22730, 22728, 22729] | 0.8 |
829 | 14895 | {23209, 23681, 23380, 22910, 23275, 22726, 231... | [22178, 47566, 22423, 84978, 82486] | 0.4 |
1270 | 16362 | {23355, 22111, 23131, 21818, 21819, 21821, 235... | [48138, 22180, 22111, 84946, 23026] | 0.2 |
1885 | 18221 | {22423, 82613D, 22092, 21210, 22093, 82613C, 2... | [22423, 22558, 47566, 84879, 84077] | 0.2 |
1770 | 17838 | {23226, 84992, 23275, 84991, 22571, 22155, 218... | [48138, 22993, 21166, 48194, 22720] | 0.0 |
출처 : 이것이 데이터 분석이다.
'이것이 데이터분석이다 with 파이썬' 카테고리의 다른 글
이것이 데이터 분석이다 wiht 파이썬 ch4-2(강남역 맛집 리뷰로 알아보는 감성 분류) (0) | 2021.05.20 |
---|---|
이것이 데이터 분석이다 with 파이썬ch2-1,2( 나무위키 최근 변경 페이지 키워드 분석하기) (1) | 2021.05.19 |
이것이 데이터 분석이다 with 파이썬 ch4-1(타이타닉 생존자 가려내기) (0) | 2021.04.16 |
이것이 데이터 분석이다 with 파이썬 ch3-3(미래에 볼 영화의 평점 예측하기) (0) | 2021.04.15 |
이것이 데이터 분석이다 with 파이썬 ch3-2(비트코인 시세 예측하기) (0) | 2021.04.14 |